기업의 더 효율적인 소프트웨어
선택을 위한 17년 지원 경험
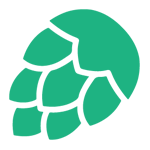
Hopsworks
Hopsworks은(는) 무엇인가요?
Hopsworks는 업계 최초의 ML용 기능 스토어를 기반으로 규모에 맞게 기계 학습(ML) 파이프라인을 개발하고 운영하기 위한 오픈 소스 엔터프라이즈 플랫폼입니다. Kubernetes 클러스터 관리 방법을 배울 필요 없이 Jupyter 노트북 및 Conda를 사용하여 Python에서 데이터를 추출하고 모델을 개발하는 것부터 프로덕션 품질의 종단 간 ML 파이프라인을 실행하는 것까지 쉽게 진행할 수 있습니다.
Hopsworks은(는) 누가 사용하나요?
관리되는 기능 스토어가 필요한 클라우드 기반 데이터 과학 팀을 대상으로 합니다. 기능 관리, 모델 학습, 제공 및 모니터링까지 전체 ML 수명 주기에 대해 확장 가능한 AI 플랫폼이 필요한 온프레미스 사용자를 대상으로 합니다.
Hopsworks에 대해 확실하지 않으세요?
인기 있는 대안 제품과 비교
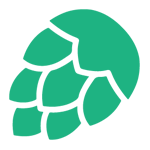
Hopsworks
Hopsworks 리뷰
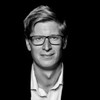
Skilled challenger to our Teradata suit
장점:
Hopsworks keeps us as a big organisation to be on our toes, in terms of what is possible and how fast it can be implemented. Nice to have options of on-premise and cloud, and extremely fast to add new libraries and other custom made wished from the data scientists.
단점:
Sometimes as it's rather flexible and fast to implement it can be hard to place it and understand it's belonging in our overall data architecture.
Hopsworks
주석: We recently analysed terabytes of cancer sequencing data and estimated what proportions of the cancers might be preventable in the future by vaccination. The paper is about to publish any day now and this study would have been impossible without Hopsworks.
장점:
As a data scientist, I am mostly focusing on developing big data processing pipelines as well as feature engineering for which Hopsworks is probably one of the best platforms. It makes it very easy to run Spark or PySpark applications to process vast amount of data with available resources. Installing python libraries for Jupiter notebook is also quite straightforward which solves many painful problems for a data scientist.
단점:
One thing I would try to improve is to get better visibility of the logs after a job is completed.
Hopsworks trial review
장점:
- project oriented dat plateform - huge set of data analytics features (feature store, automl, lakehouse, kafka, spark, flink, ....) - devsecops oriented product (security, stretched cluster, model serving, scm, ...) - on-premise, cloud , multi-cloud (potential) and hybrid-cloud (potential) platform - easiness of use - european company - open source based product - gdpr compliancy - deep learning compliancy (gpu, tpu(?), pytorch, tensorflow) - openess (databricks, sagemake, ..., connectors) - ...
단점:
- Hudi instead of deltalake - lack of connection between deltalake (as provided with Hudi) and the feature store - unability to use the ELK or influxdb included tools - lack of connectors with AzureML, driveless ai, powerbi, azure datablob storage, snowflake, ... - lack of managed platform on azure or gcp as the one provided for aws - how to handle staging environment especially for the data sharing? - ability to deploy on a kubernetes environment outside hopsworks - R connection along with Python - difficulties to knwo the arguments for choosing hopsworks instead of dtabacricks, azureml, sagemaker, .... - lack of prepackaged, ready-to-use managed platform on an appliance for including intot a private datacenter - I don't know the pricing policy, and I'm not capable of comaring it with the competitor ones - no graphical etl-like tools enabling to create quickly a data engineering process and deploy it (à la dremio or dataiku)